Research
The VIDA research group goes beyond responding to the latest trends by being the trailblazers in setting new trends in computer vision, medical imaging, artificial intelligence, machine learning, and data science. Our collaborative team prides itself on research that is cutting-edge, multidisciplinary, and transformative. Below, you can find some of the VIDA group's current and past project along with published papers related to each project.
Current Projects
*[numbers in front of citations represent paper numbers on the VIDA group's publication page]
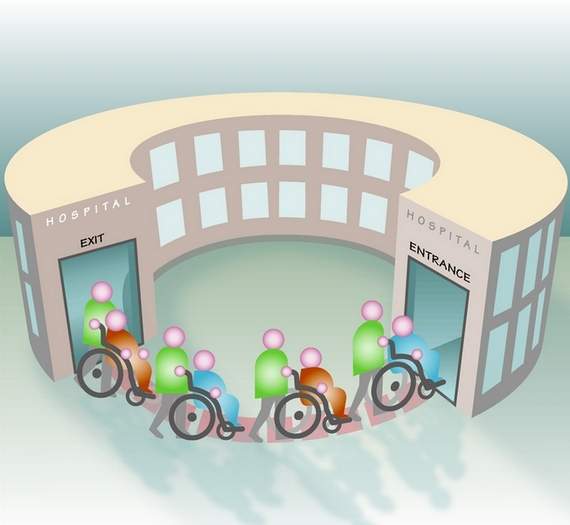
Emergency department (ED) readmissions within 30 days are a vital measure for healthcare providers, as they significantly impact both patient outcomes and hospital financial performance. Reducing unplanned readmissions is a critical goal for hospitals, as it helps ease the burden on healthcare systems while improving the quality of care delivered. Social determinants of health (SDoH)—such as housing stability, education, and food security—play a pivotal role in shaping health outcomes, especially among vulnerable populations. Hospitals document these factors in patients' medical records and connect individuals to relevant community resources to address their needs. Community Health Workers (CHWs) are central to this effort, acting as a vital link between clinical care and community support through culturally tailored assistance and resource coordination.
For more information on the Scholar Project, visit: Scholar Project Page
Related Papers:
[209] Hernandez N., Karam K., Baugh N., Musale S., Moses A.P., Raicu DS., Furst JD., McCabe K. and Tchoua R.,
"Leveraging community health workers for predicting emergency department readmissions"
. International Journal of Semantic Computing, 2024
[208] Karam K., McCabe K., Tchoua R.,
"Leveraging community health workers for predicting emergency department readmissions"
. Fifth International Conference on Transdisciplinary AI (TransAI), 2023
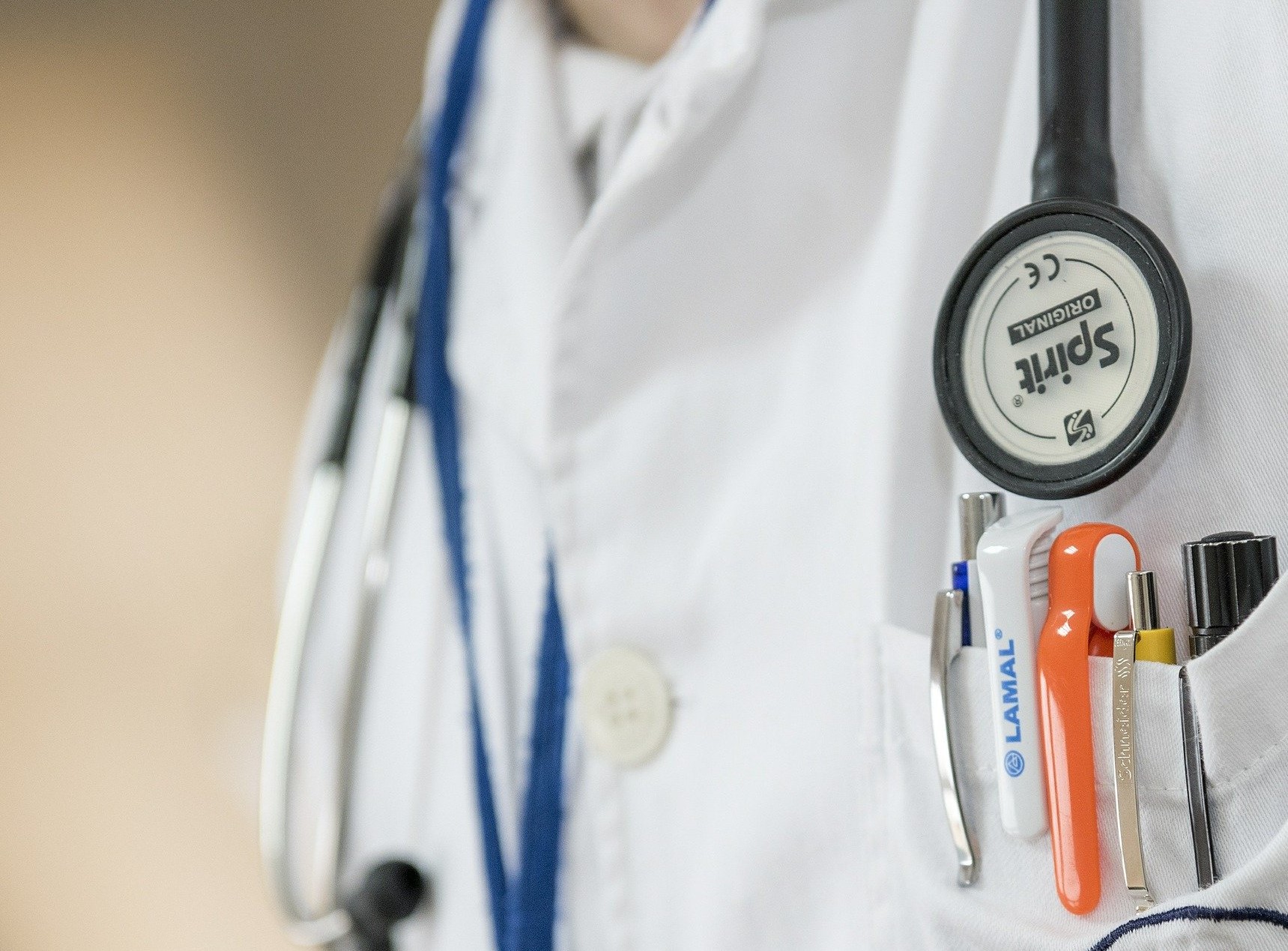
Primary care clinics play a vital role in identifying disease at early stages, providing continuous comprehensive care to the patients, and overall providing better healthcare facilities. In such primary care settings, patient-physician communication plays a crucial role in patient satisfaction and overall health outcomes. With advances in technology, primary health care centers have adopted Electronic Health Record (EHR) systems in the clinical care settings. Although EHRs have been adopted to improve medication management and documentation of patient health records, EHRs seem to affect negative effects on the physicians. The overall communication between the patient and the physician seems to be affected. This project aims to understand the impact of healthcare technology on both patients and doctors in primary clinical care settings. The current stage of the project includes identifying physician's gaze using computer vision and deep learning techniques. The data used in the project are recorded video interactions between the physician and the patient.
Related Papers:
[180] Tan T., Montague E., Furst JD., and Raicu DS., "Robust Physician Gaze Prediction Using a Deep Learning Approach". The 20th IEEE International Conference on BioInformatics And BioEngineering (BIBE 2020), Virtual Conference, October 26-28, 2020
[179] Govindaswamy AG., Montague E., Raicu DS., Furst JD., "CNN as a feature extractor in gaze recognition" , In 2020 3rd Artificial Intelligence and Cloud Computing Conference (AICCC 2020), Kyoto, Japan, December 18–20
[178] Govindaswamy AG, Montague E, Raicu DS, Furst J. "Predicting physician gaze in clinical settings using optical flow and positioning" . In 2020 35th International Conference on Image and Vision Computing New Zealand (IVCNZ), November 25, 2020
[172] Tan T., Montague E., Furst J., Raicu D. "Developing parameters for a technology to predict patient satisfaction in naturalistic clinical encounters" . In: Duffy V. (eds) Digital Human Modeling and Applications in Health, Safety, Ergonomics and Risk Management. Posture, Motion and Health. International Conference on Human Computer Interaction (HCII 2020), Lecture Notes in Computer Science, vol 12198. Springer, Cham.
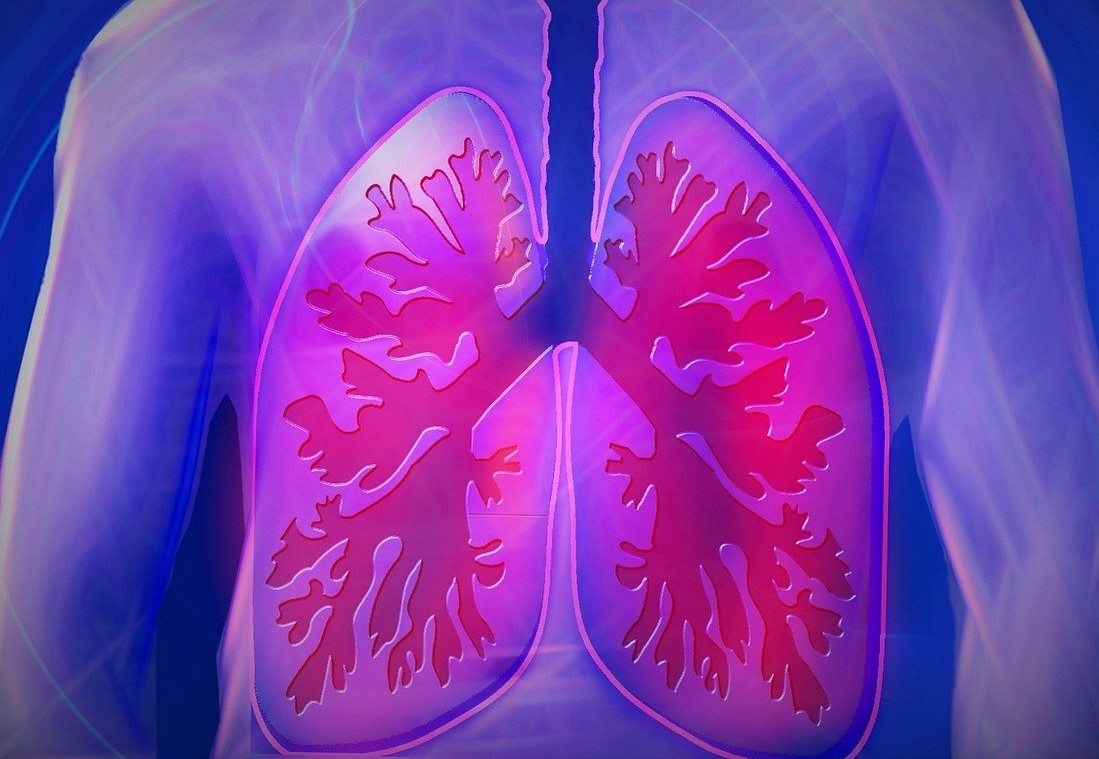
Accurate diagnosis of lung lesions in computed tomography (CT) depends on many factors, including the radiologists’ ability to detect and correctly interpret these lesions. Computer-aided diagnosis (CAD) systems can be used to measurably increase the accuracy of radiologists in this task. Various CAD systems have been developed over the years for the detection and classification of pulmonary nodules. Most of these systems mimic domain knowledge in order to extract image content and use a comparison with ground truth for evaluation. However, these systems work in an algorithmic fashion that is only tenuously related to human perception and characterization of image features. In the image retrieval community, this is known as the semantic gap problem – the lack of coincidence between the quantitative information that may be extracted computationally from the image data and the visual interpretation of this data by human observers. The goals of this research study are 1) to establish the link between computer-based image features of lung nodules in CT scans and visual descriptors defined by human experts in the Lung Image Database Consortium (LIDC) terminology and 2) integrate these links into content-based lung nodule image retrieval (CBIR) systems. The rationale is that such systems will permit 1) reference and teaching by providing retrieval of cases similar to a given image and 2) decision support by retrieving cases with similar features and providing assisted diagnosis based on known status in retrieved cases.
Related Papers:
[162] Yu Y., Wang Y., Furst JD., Raicu DS., "Identifying diagnostically complex cases through ensemble learning". 2019 International Conference on Image Analysis and Recognition (ICIAR), Waterloo, Canada, August 27-29, 2019
[161] Yu Y., Weddell R., Furst JD., Raicu DS. "Utilization of Instance Hardness in Multiple Annotations Active Learning". ACM SIGKDD Data Collection, Curation, and Labeling for Mining and Learning Workshop, Anchorage, Alaska, August 4-8, 2019
[174] Qiu B., Furst J., Rasin A., Tchoua R., Raicu D. "Learning latent spiculated features for lung nodule characterization". the IEEE Engineering in Medicine and Biology Society, Montreal, Canada, July 20, 2020.
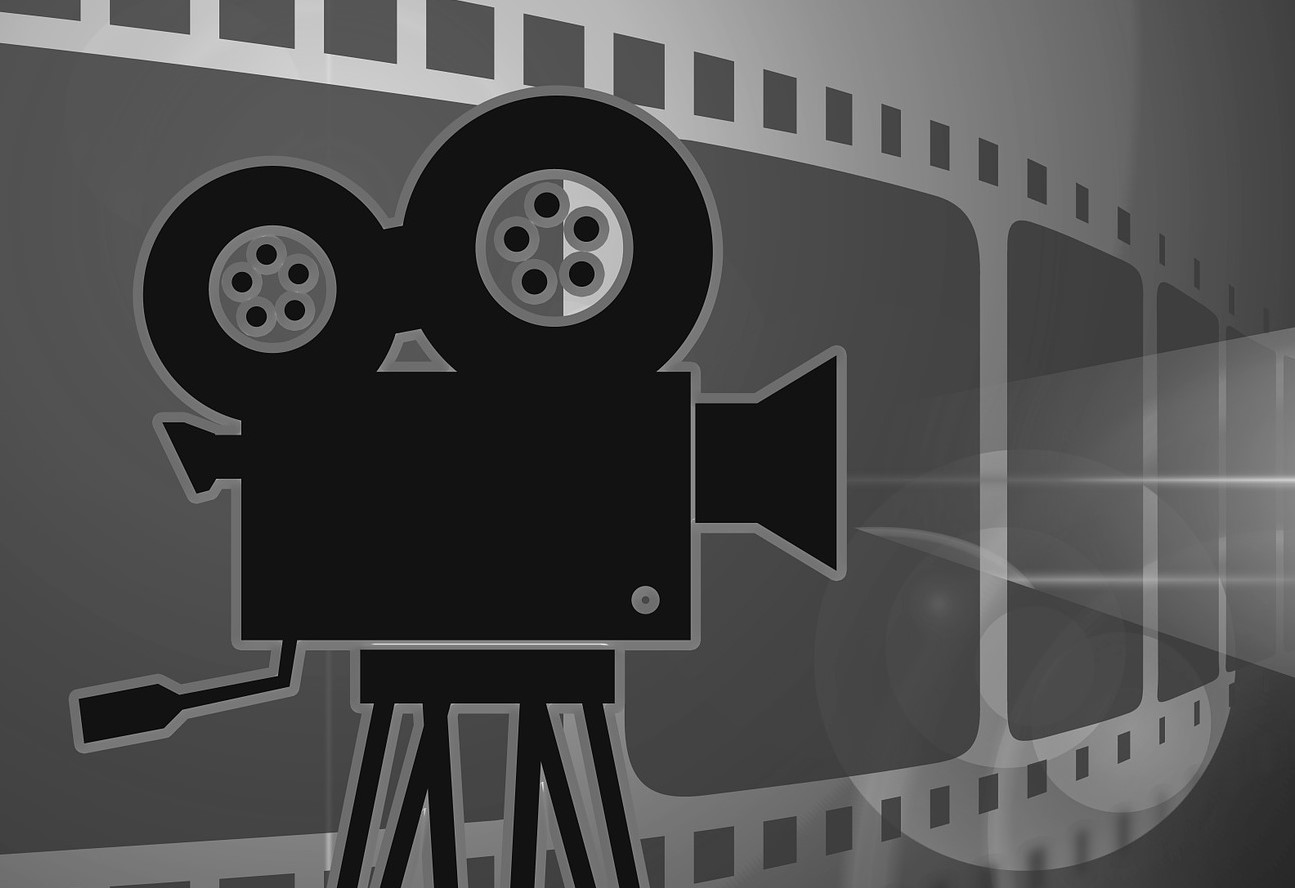
Performing video analysis for activity recognition presents challenges beyond classification, including obtaining class labels and temporal segmentation. One such challenge is precisely annotating a video with class labels having the exact start and end points of an activity; a difficult task for a human to perform, especially when one relevant activity immediately follows another. Furthermore, the task of annotating a video at any level of precision can quickly become tedious, impacting the attentiveness of the annotator, resulting in annotation errors. Human-in-the-loop methods that simply ask for a class label at different points during the video will have similar problem with the precision of start and end points of activity as well as the potential increased cognitive workload when the clip to be labeled is shown to the annotator and more contextual information is needed to determine the correct label. The purpose of this research is to investigate the integration of signal processing methods for motion feature extraction (e.g. speed/velocity of a subject as observed in the video) and analysis (e.g. speed shape patterns) into temporal video segmentation and activity labeling. The hypothesis is that motion-related shape patterns are key indicators of certain types of activities, provide cues of where these activities begin and end, and consequently, contribute to the temporal segmentation of video data and activity recognition. We hypothesize that, when these methods are applied to an iterative human-in-the-loop process, the system and human will converge on the ‘true’ set of class labels. In addition to more accurate annotations, the efficiency of the annotation will be also improved. Rather than annotating each video frame, an annotator will be presented with video sequences where each sequence contains a certain shape motion pattern (e.g. turn, reversal).
Related Papers:
[158] Piane J., Wang Y., Cheung T., Kim H., Furst JD., Raicu DS., "Augmenting Frame-based with Window-based Features for C. elegans Movement Classification", IEEE EMBS International Conference on Biomedical & Health Informatics, Chicago, IL, May 19-22, 2019
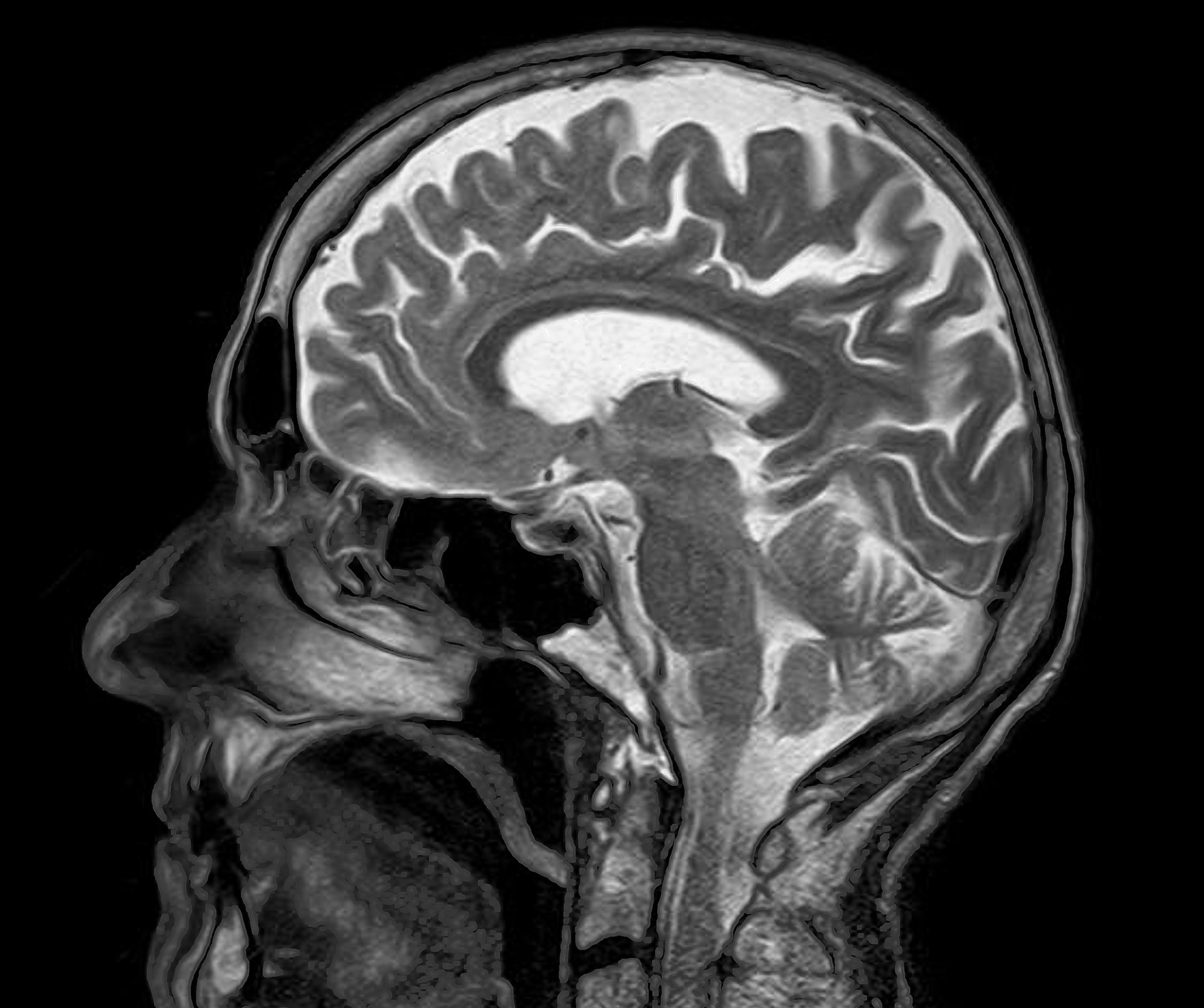
Radiology teaching files serve as a reference in the diagnosis process and as a learning resource for radiology residents. There are many public teaching file data sources available online; private in-house teaching file repositories are further maintained in most hospitals. Teaching files include both text and images with structural variations even within the same data repository. Moreover, the native interfaces in existing repository have a very limited search capability. The Integrated Radiology Image Search (IRIS) engine is designed to combine publicly available data sources and in-house teaching files in a unified resource. IRIS provide text-based, image-based, and hybrid (text + image) search functionality.
Related Papers:
[175] Deshpande P., Rasin A., Tchoua R., Furst JD., Antani S, and Raicu DS , “Enhancing Recall Using Data Cleaning for Biomedical Big Data”. IEEE 33rd International Symposium on Computer Based Medical Systems (CBMS), July 28-30, 2020, CBMS 2020.
[164] Deshpande P., Rasin A., Cao F., Yarlagadda S., Brown ET., Furst JD., Raicu DS., "Multimodal Ranked Search over Integrated Repository of Radiology Data Sources". Knowledge Discovery and Information Retrieval, Vienna, Austria, September 17-19, 2019
[163] Deshpande P., Rasin A., S. Jun, Kim S., Brown ET., Furst JD., Montner S., Armato SG., Raicu DS., "Ontology-based Radiology Teaching Files Summarization, Coverage, and Integration". Journal of Digital Imaging, JDI, 2019
[151] Deshpande P., Rasin A., Brown ET., Furst JD., Montner S., Armato SG., Raicu DS., "Augmenting Medical Decision Making With Text-Based Search of Teaching File Repositories and Medical Ontologies: Text-Based Search of Radiology Teaching Files". International Journal of Knowledge Discovery in Bioinformatics (IJKDB), 8(2), 18-43
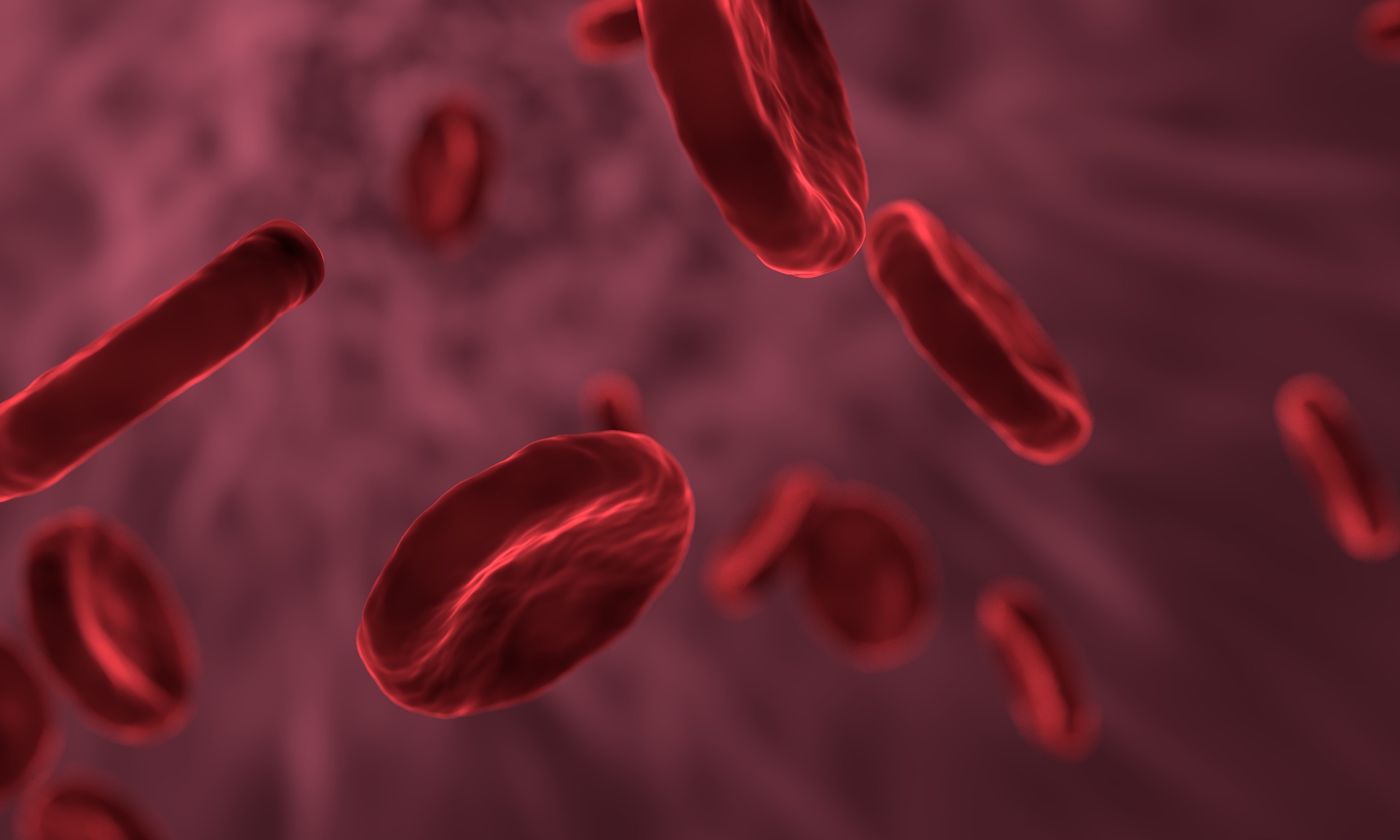
This project explores data science in the context of predicting a disease with no know etiology: Myalgic encephalomyelitis/chronic fatigue syndrome. This project includes blood protein data from over 100 young adult participants at three time periods: baseline (healthy), during mono (all participants suffered through an infection) and then six months post-mono, when participants had either fully recovered or had developed ME/CFS. This study differs from many predictive studies in that the relevant data are correlations: blood proteins are known to work together, and so the correlations predict better than raw data. This requires new algorithms to generate labels for partipants.
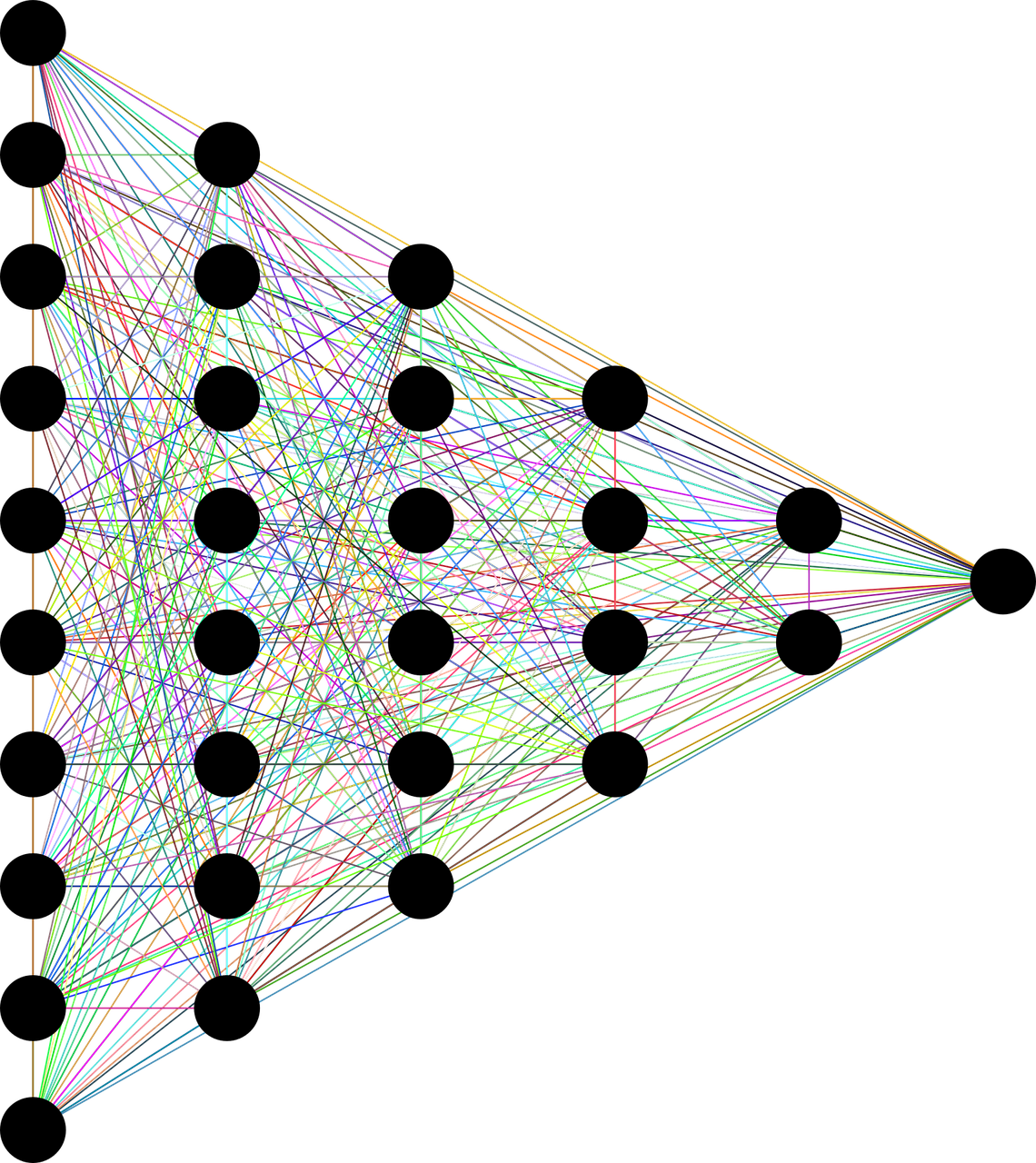
Convolutional neural nets are typically composed of two major components: a convolutional network that generates an intermediate representation from image data; and a dense neural network, that learns labels from the intermediate representation. Unfortunately, dense neural networks are black box learners that offer no intuition about the learning and have two significant training drawbacks: 1) the require large datasets to learn robustly and 2) require significant time to learn the millions of parameters often required. This project replaces the dense neural net with a k-nearest-neighbor learner that is fast, intuitive, and can learn from small datasets.
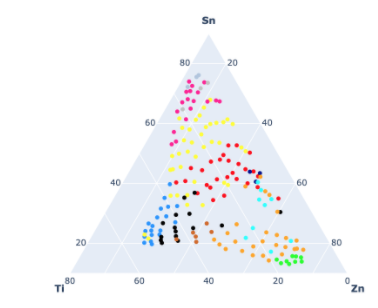
Data science is intrinsically inter-disciplinary; however, end-users of machine learning models are not always trained data scientists. On the other hand, it is crucial that these models be infused with domain knowledge in order to increase explainability and trust in their output. Our goal in this project is to provide domain-aware confidence scores and enable domain experts to interact with, and guide the clustering. Our hypothesis is that given confidence scores, end-users will be more willing to trust and adopt machine learning models. We test this hypothesis with materials informatics, a field that has the potential to greatly reduce time-to-market and development costs for new materials as it leverages machine learning and large datasets for targeted design. For example, automated phase-mapping seeks to discover samples of materials mixture with similar structure. This is challenging because measurements per samples far exceed the number of samples to clustering making it difficult to interpret and generalize. Towards our goal, we are building a dashboard comparing clustering methods. We envision that scientist will not only be able to assess confidence scores but also interact with results, merging and splitting clusters, guiding the discovery process. We describe the signals in terms of peaks and other interpretable features; we compare and contrast multiple clustering techniques and provide several visualization options (e.g., layered graphs, samples closest to and farthest from centroids) to assist domain experts through the clustering of this complex data.
Related Papers:
Bunn, Jonathan Kenneth, Jianjun Hu, and Jason R. Hattrick-Simpers. "Semi-supervised approach
to phase identification from combinatorial sample diffraction patterns."; Jom 68, no. 8 (2016):
2116-2125.
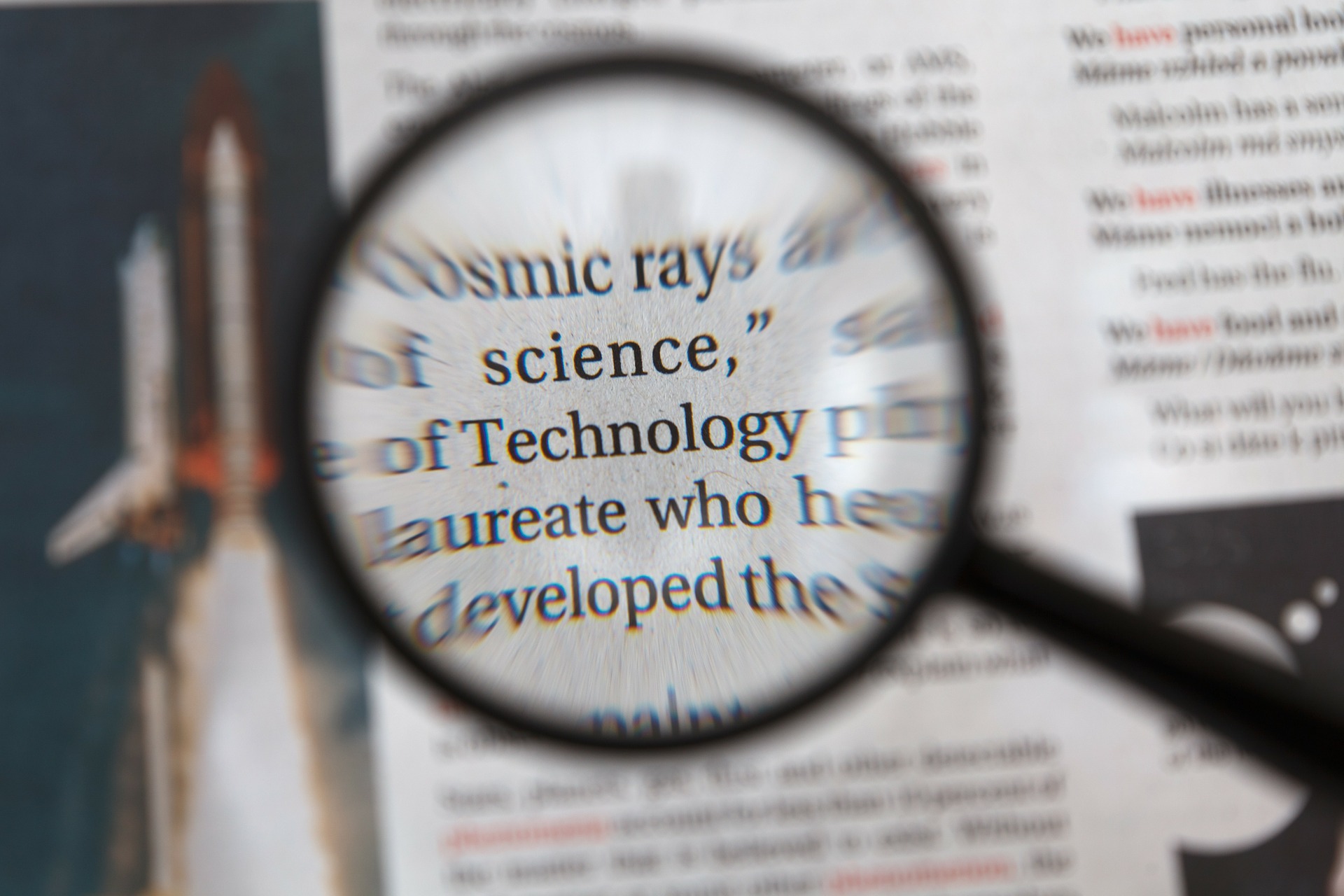
Extracting scientific facts from unstructured text is difficult due to challenges specific to the ambiguity of the language, the complexity of the scientific named entities and relations to be extracted. This problem is well illustrated through the extraction of polymer names and their properties. Even in the cases where the property is a temperature, identifying the temperature’s polymer name may require expertise due to the use of acronyms, synonyms, complicated naming conventions and by the fact that new polymer names are being “introduced” to the vernacular as polymer science advances. While there exist domain-specific machine learning toolkits that address these challenges, perhaps the greatest challenge is the lack of—time-consuming, error- prone, and costly—labeled data to train these machine learning models. We have previously worked on Ensemble Labeling for Scientific Information Extraction(ELSIE) to identify sentences that contain the information to be extracted as a first step towards extracting the target information. We have extended ELSIE to identify important paragraphs as the information was sometimes scattered across sentences. Through ELSIE-Blob we are now able to extract more important sentences from publications. The next step in this project is to extract scientific facts from relevant sentences.
Related Papers:
Murphy, E., Rasin, A., Furst, J., Raicu, D., & Tchoua, R. (2021, June). Ensemble labeling towards scientific information extraction (ELSIE). In International Conference on Computational Science (pp. 750-764). Springer, Cham.
Tchoua, R. B., Chard, K., Audus, D. J., Ward, L. T., Lequieu, J., De Pablo, J. J., Foster, I. T. (2017, October). Towards a hybrid human-computer scientific information extraction pipeline. In 2017 IEEE 13th international conference on e-Science (e-Science) (pp. 109-118). IEEE.
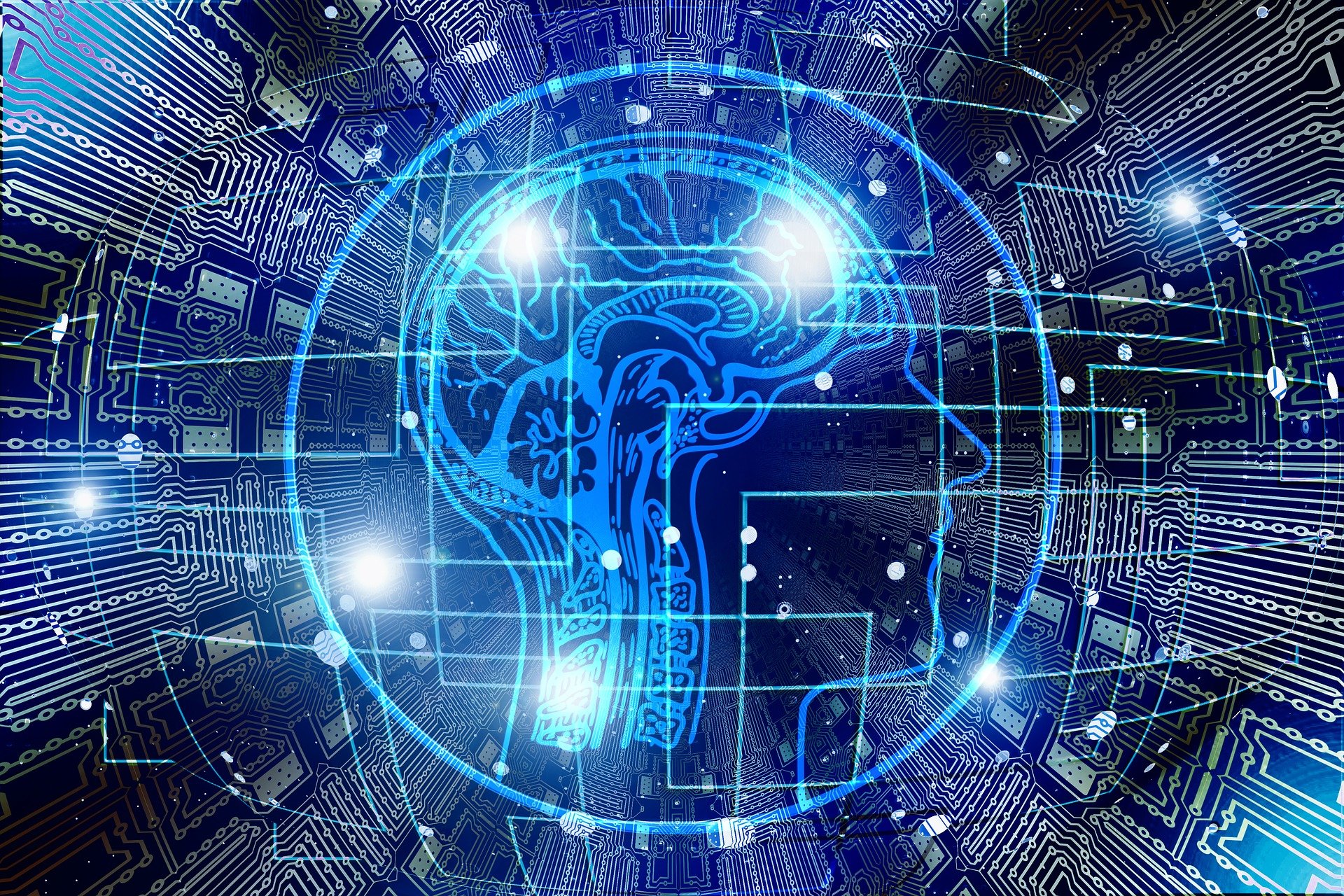
There is significant effort to increase the adoption of Deep Neural Networks (DNN) in fields such as criminal justice and medicine. Yet, DNNs commonly fall short in these applications due to their “black box” nature. DNNs generally output a single value for classification accuracy of a data set, creating a lack of transparency and interpretation. DNN research often focuses on achieving a higher classification accuracy, but this does not apply well to data sets that are inherently difficult to classify. In such cases, scientists not only expect a prediction but also an explanation and/or a measure of confidence per prediction. While several machine learning algorithms address this issue, this topic is still the subject of intense research. In this project, we propose a novel uncertainty metric based on clustering and data similarity learned through a Siamese Convolutional Neural Network (SCNN). Rather than using the silhouette coefficient as a measure for clustering, we use it to independently provide an uncertainty score and supplement each prediction. These uncertainty scores serve to better inform the end-user's decision, in our case an expert application scientist.
Related Papers:
[174] Qiu, B., Furst, J., Rasin, A., Tchoua, R., & Raicu, D. (2020, July). Learning latent spiculated
features for lung nodule characterization. In 2020 42nd Annual International Conference of the IEEE Engineering in Medicine & Biology Society (EMBC) (pp. 1254-1257). IEEE.
Past Projects
A challenge in neuroscience is understanding the genetic bases of behavior; traditionally, the nematode Caenorhabditis elegans is used as a model organism for neural studies due to its simple neural structure and well known connectome. Our study aims to identify genes that modulate food related behaviors in nematodes by quantifying behavioral differences in mutant strains. In order to empirically quantify nematode behavior, we have developed methods for recording and tracking nematodes over long periods of time, as well as algorithms for extracting and analyzing information from the observational data.
Advanced form of age-related macular degeneration (AMD) is a major health burden that can lead to irreversible vision loss in the elderly population. For early preventative interventions, there is a lack of effective tools to predict the prognosis outcome of advanced AMD because of the similar visual appearance of retinal image scans in the early stage and the variability of prognosis paths among patients. The existing prognosis models have several limitations: First, previous studies assume constant time intervals between doctor visits; however, in real world clinical settings, the visits may happen at irregular time intervals. The assumption of constant time intervals will lead to over-optimistic prediction results on specific training data sets while failing to produce generalizable results on new patient data sets. Second, current studies only predict one form of advanced AMD form at a time. Third, computer-based prognosis results are typically not validated on new patients and therefore, it is difficult to evaluate the generalizability of the proposed approaches. Lastly, there is a lack of interpretability of the models and explainability of how a computer-based prognosis determination has been made. The overall objective for this project is to design, develop, and evaluate AMD prognosis prediction models that can detect most relevant images containing AMD biomarkers, manage unevenly spaced sequential optical coherence tomography (OCT) images and predict all advanced AMD forms that can help with the interpretation and explainability of computer-aided prognosis models.
Focused on reducing the semantic gap by investigating computer-based similarity measures and image features that are close to the human perception of similarity and encoded the visual content of an image similarly to the human vision.
Proposed the development of a full-featured and scalable IE system capable of converting free-text radiology reports to a structured format.
Proposed: 1) a novel automatic mass segmentation method for identifying the contour (boundary) of a mass from a suspicious region (ROI) in a mammogram; 2) A multiple segmentation approach which builds multiple weak segmentors for each ROI image by applying a set of different image enhancements for mass segmentation; and 3) An ensemble approach where multiple base-level classifiers are built as experts from different perspectives to predicate the class probabilities; then, utilizing another learning algorithm, a meta-level classifier combines the diagnoses to generate the final diagnosis for a suspicious mass.
Approached a patent database and its citations with theoretical models applied from statistical analysis and physics. Using the clustering techniques of complex networks, we searched for patterns of relationships between patents of different categories.
Argonne National Laboratory (ANL) funded research work within the context of the Department of Homeland Security (DHS) microbial forensics programs toward the development of statistically-based experimental designs, microarray image analysis and decision tools for the analysis of genotyping and single nucleotide polymorphism microarrays.